Master’s of Artificial Intelligence
Gain the Technical Skills to Stand Out in the World of AI
Artificial intelligence is poised to drive the next generation of global innovation. The need for skilled AI professionals is greater than ever, with 97 million new AI-related jobs expected globally over the next two years.6 The UT Austin online master’s degree in AI prepares you to stand out in this fast-growing field through one of the first AI master’s programs available 100% online.
Learn AI Insights from
Expert UT Austin Faculty
Be Among the First to Graduate with an Online Master’s in AI
Affordable, Advanced Degree Priced at $10,000+ Fees7
Curriculum
The online AI master’s coursework covers a range of highly sought after skills to prepare you to lead AI innovations across a variety of industries, from engineering and medicine to finance and project management.
You will study reasoning under uncertainty, ethics in AI, case studies in machine learning, and more from some of UT Austin’s world-class faculty and collaborate with fellow students.
Featuring on-demand lectures and weekly release schedules, these asynchronous, instructor-paced courses are designed to be accessed on your schedule, from wherever you are.
6World Economic Forum, The Future of Job Report 2020-2025. Accessed January 2023.
7International student fees and late registration fees may apply.
Courses
Ethics in AI (required)
+
nine elective courses
=
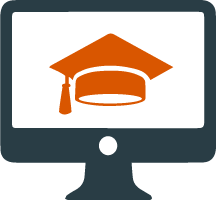
Ten Courses
The online master’s degree in artificial intelligence is a 30-hour program consisting of 3 hours of required courses and 27 hours of electives. Each course counts for 3 credit hours and you must take a total of 10 courses to graduate. It is recommended that MSAI students complete the required and foundational courses in the beginning of their program before completing their elective courses.
Required and Foundational Courses
This class covers advanced topics in deep learning, ranging from optimization to computer vision, computer graphics and unsupervised feature learning, and touches on deep language models, as well as deep learning for games.
Part 1 covers the basic building blocks and intuitions behind designing, training, tuning, and monitoring of deep networks. The class covers both the theory of deep learning, as well as hands-on implementation sessions in pytorch. In the homework assignments, we will develop a vision system for a racing simulator, SuperTuxKart, from scratch.
Part 2 covers a series of application areas of deep networks in: computer vision, sequence modeling in natural language processing, deep reinforcement learning, generative modeling, and adversarial learning. In the homework assignments, we develop a vision system and racing agent for a racing simulator, SuperTuxKart, from scratch.
What You Will Learn
- About the inner workings of deep networks and computer vision models
- How to design, train and debug deep networks in pytorch
- How to design and understand sequence
- How to use deep networks to control a simple sensory motor agent
Syllabus
- Background
- First Example
- Deep Networks
- Convolutional Networks
- Making it Work
- Computer Vision
- Sequence Modeling
- Reinforcement Learning
- Special Topics
- Summary
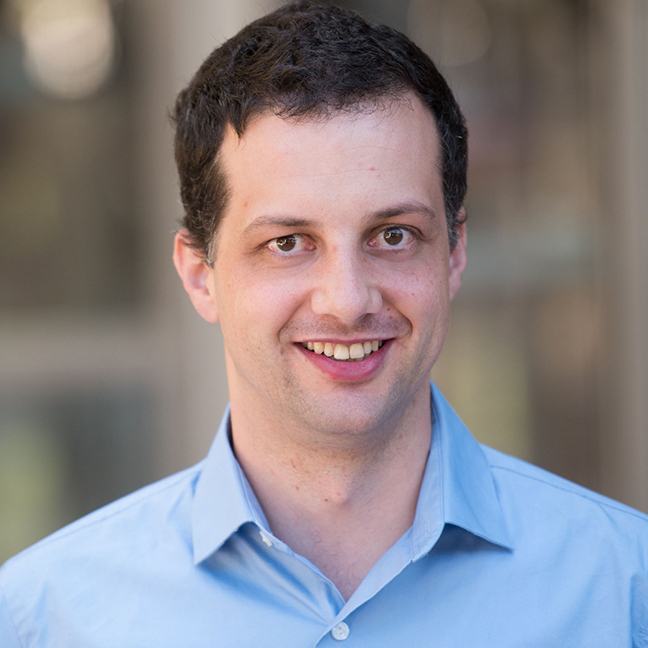
Assistant Professor, Computer Science
Artificial intelligence (AI) is both a product of and a major influence on society. As AI plays an increasingly important role in society, it is critical to understand both the ethical factors that influence the design of AI and the ethical dimensions of the impacts of AI in society. The goal of this course is to prepare AI professionals for the important ethical responsibilities that come with developing systems that may have consequential, even life-and-death, consequences. Students first learn about both the history of ethics and the history of AI, to understand the basis for contemporary, global ethical perspectives (including non-Western and feminist perspectives) and the factors that have influenced the design, development, and deployment of AI-based systems. Students then explore the societal dimensions of the ethics and values of AI. Finally, students explore the technical dimensions of the ethics and values of AI, including design considerations such as fairness, accountability, transparency, power, and agency.
Students should take this course to prepare them for the ethical challenges that they will face throughout their careers, and to carry out the important responsibilities that come with being an AI professional. The ethical dimensions of AI may have important implications for AI professionals and their employers. For example, the release of unsafe or biased AI-based systems may cause liability issues and reputational damage. This course will help students to identify design decisions with ethical implications, and to consider the perspectives of users and other stakeholders when making these ethically significant design decisions.
Students who perform well in this class will be positioned to take on a leadership role within their organizations and will be able to help guide and steer the design, development, and deployment of AI-based systems in ways that benefit users, other stakeholders, their organizations, and society. The knowledge and skill gained through this course will benefit students throughout their careers, and society as a whole will benefit from ensuring that AI professionals are prepared to consider the important ethical dimensions of their work.
What You Will Learn
- You will learn about the history of AI and the ethical challenges that arise from AI
- You will learn about a wide range of ethical theories and learn to apply them to the ethics of AI
- You will learn about efforts to develop principles for the design of ethical AI
Syllabus
- Week 1: Introduction
- Week 2: Indian Ethics/Classical Chinese Ethics/Babbage’s Engines
- Week 3: Buddhist Ethics/Islamic Ethics/Dartmouth Conference on AI
- Week 4: Kantian Ethics/Consequentialism/Deep Blue
- Week 5: Distributive Justice/Virtue Ethics/Watson
- Week 6: Ethics of Care/Ubuntu/Autonomous Cars
- Week 7: Human Values/Value Sensitive Design
- Week 8: Codes of Ethics
- Week 9: AI Ethics Guidelines
- Week 10: Fairness
- Week 11: Accountability
- Week 12: Transparency
- Week 13: Power
- Week 14: Agency
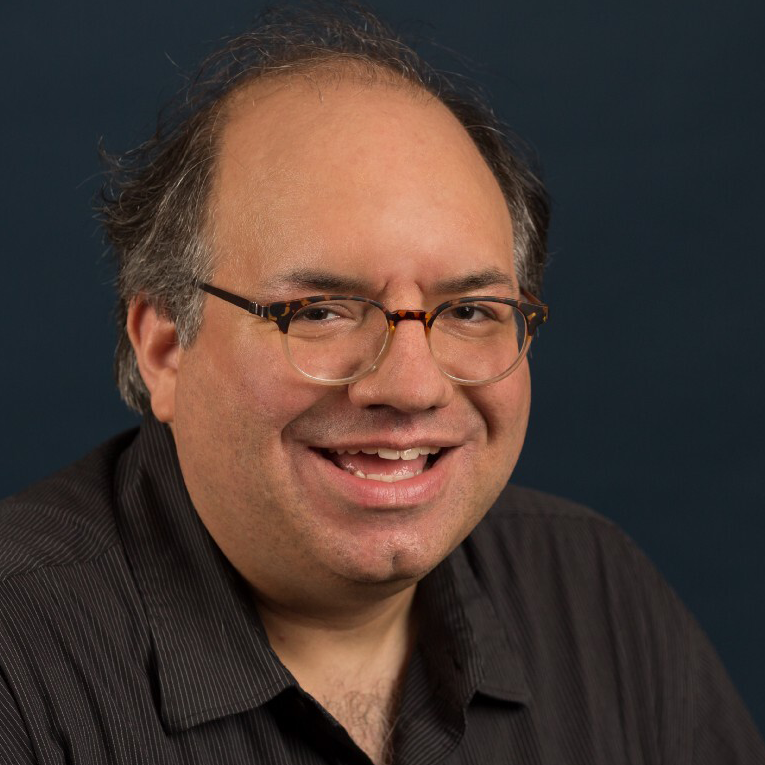
Professor, School of Information
This course focuses on core algorithmic and statistical concepts in machine learning.
Tools from machine learning are now ubiquitous in the sciences with applications in engineering, computer vision, and biology, among others. This class introduces the fundamental mathematical models, algorithms, and statistical tools needed to perform core tasks in machine learning. Applications of these ideas are illustrated using programming examples on various data sets.
Topics include pattern recognition, PAC learning, overfitting, decision trees, classification, linear regression, logistic regression, gradient descent, feature projection, dimensionality reduction, maximum likelihood, Bayesian methods, and neural networks.
What You Will Learn
- Techniques for supervised learning including classification and regression
- Algorithms for unsupervised learning including feature extraction
- Statistical methods for interpreting models generated by learning algorithms
Syllabus
- Mistake Bounded Learning (1 week)
- Decision Trees; PAC Learning (1 week)
- Cross Validation; VC Dimension; Perceptron (1 week)
- Linear Regression; Gradient Descent (1 week)
- Boosting (.5 week)
- PCA; SVD (1.5 weeks)
- Maximum likelihood estimation (1 week)
- Bayesian inference (1 week)
- K-means and EM (1-1.5 week)
- Multivariate models and graphical models (1-1.5 week)
- Neural networks; generative adversarial networks (GAN) (1-1.5 weeks)
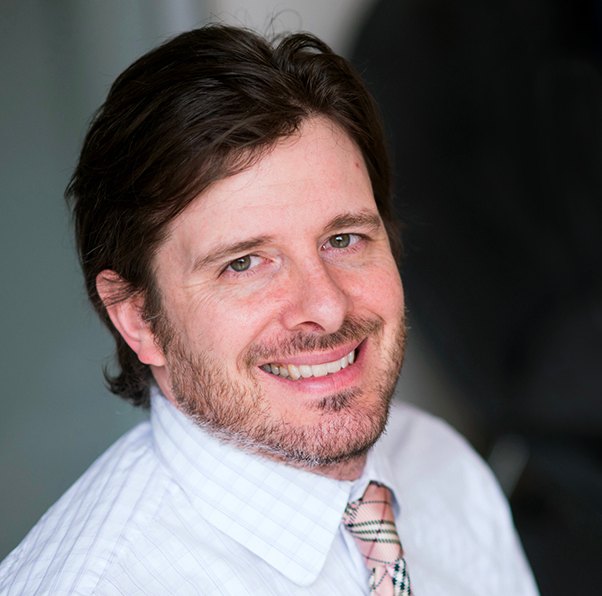
Professor, Computer Science
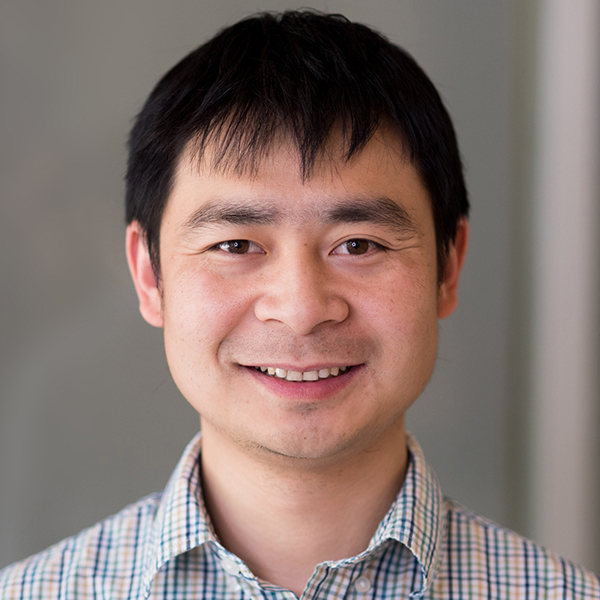
Assistant Professor, Computer Science
We will investigate how to define planning domains, including representations for world states and actions, covering both symbolic and path planning. We will study algorithms to efficiently find valid plans with or without optimality, and partially ordered, or fully specified solutions. We will cover decision-making processes and their applications to real-world problems with complex autonomous systems. We will investigate how in planning domains with finite state lengths, solutions can be found efficiently via search. Finally, to effectively plan and act in the real world, we will study how to reason about sensing, actuation, and model uncertainty. Throughout the course, we will relate how classical approaches provided early solutions to these problems, and how modern machine learning builds on, and complements such classical approaches.
What You Will Learn
- Defining and solving planning problems
- Planning algorithms for discrete and continuous state spaces
- Adversarial planning
- Bayesian state estimation
- Decision-making in probabilistic domains
Syllabus
- Topic 1: Planning Domain Definitions and Planning Strategies (1 week)
- Topic 2: Heuristic-Guided, and Search-based Planning (2 weeks)
- Topic 3: Adversarial Planning (2 weeks)
- Topic 4: Configuration-Space Planning/Sample-Based Planning (2 weeks)
- Topic 5: Probabilistic Reasoning/Bayesian State Estimation(2 weeks)
- Topic 7: Markov Decision Processes (1 week)
- Topic 8: Partially Observable Markov Decision Processes (1 week)
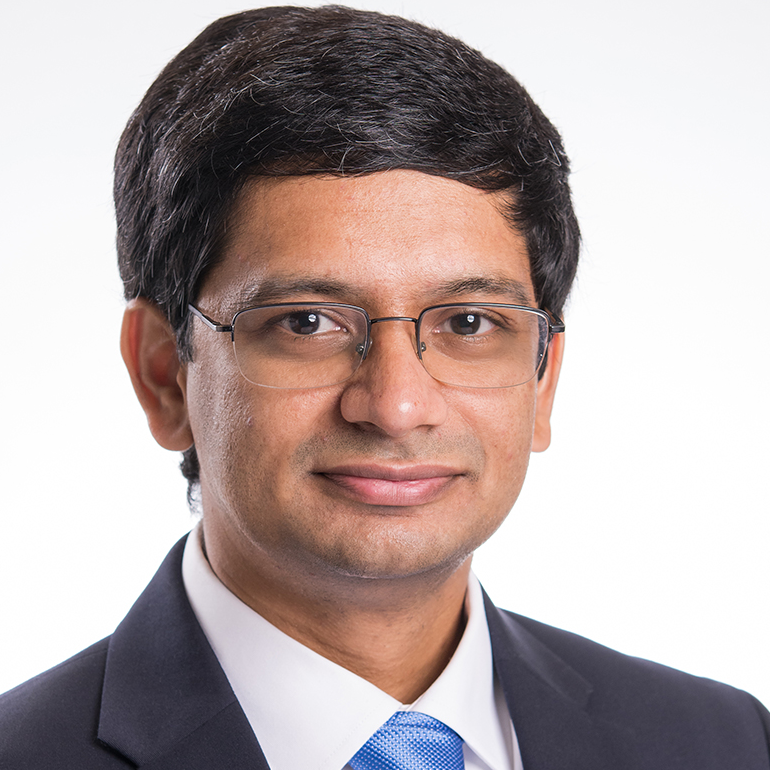
Associate Professor
This course introduces the theory and practice of modern reinforcement learning. Reinforcement learning problems involve learning what to do—how to map situations to actions—so as to maximize a numerical reward signal. The course will cover model-free and model-based reinforcement learning methods, especially those based on temporal difference learning and policy gradient algorithms. Introduces the theory and practice of modern reinforcement learning. Reinforcement learning problems involve learning what to do—how to map situations to actions—so as to maximize a numerical reward signal. The course will cover model-free and model-based reinforcement learning methods, especially those based on temporal difference learning and policy gradient algorithms. It covers the essentials of reinforcement learning (RL) theory and how to apply it to real-world sequential decision problems. Reinforcement learning is an essential part of fields ranging from modern robotics to game-playing (e.g. Poker, Go, and Starcraft). The material covered in this class will provide an understanding of the core fundamentals of reinforcement learning, preparing students to apply it to problems of their choosing, as well as allowing them to understand modern RL research. Professors Peter Stone and Scott Niekum are active reinforcement learning researchers and bring their expertise and excitement for RL to the class.
What You Will Learn
- Fundamental reinforcement learning theory and how to apply it to real-world problems
- Techniques for evaluating policies and learning optimal policies in sequential decision problems
- The differences and tradeoffs between value function, policy search, and actor-critic methods in reinforcement learning
- When and how to apply model-based vs. model-free learning methods
- Approaches for balancing exploration and exploitation during learning
- How to learn from both on-policy and off-policy data
Syllabus
- Multi-Armed Bandits
- Finite Markov Decision Processes
- Dynamic Programming
- Monte Carlo Methods
- Temporal-Difference Learning
- n-step Bootstrapping
- Planning and Learning
- On-Policy Prediction with Approximation
- On-Policy Control with Approximation
- Off-Policy Methods with Approximation
- Eligibility Traces
- Policy Gradient Methods
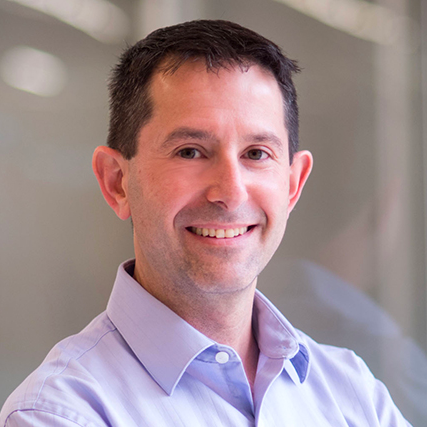
Peter Stone Instructor of Record
Professor, Computer Science
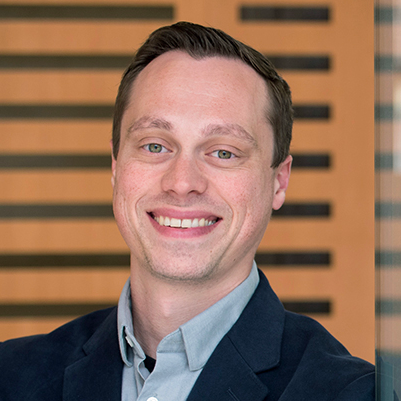
Adjunct Assistant Professor, Computer Science
Elective Courses
This course provides an in-depth exploration of the technologies behind some of the most advanced deep learning models, including diffusion models and cutting-edge models in language and computer vision. Through hands-on assignments, students will re-implement smaller versions of these models, allowing them to gain practical experience and a deep understanding of how these AI technologies function.
The course is designed to address the fact that only a small group of individuals, with significant resources, will ever train large frontier models. However, most professionals will interact with advanced AI models, either directly or indirectly. This class is valuable because it equips students with the knowledge needed to understand how these AI models work, their limitations, and how to form an accurate mental model of their capabilities. By gaining this understanding, students will be better prepared to work with and critically assess advanced AI technologies.
By the end of the course, students will be able to:
- Comprehend the inner workings of the most advanced deep learning models.
- Train and fine-tune some of these models, providing them with practical skills and hands-on experience in deep learning.
Advances in Deep Learning offers a balance between theory and application, ensuring that students leave with a robust understanding of the core technologies driving modern AI and practical experience in working with these models.
Course outline will be posted soon.
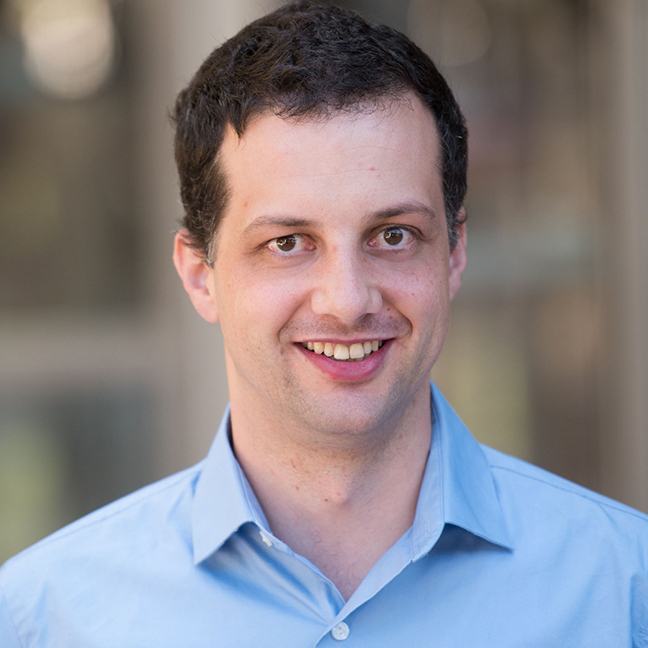
Assistant Professor, Computer Science
This course explores the major components of health IT systems, ranging from data semantics (ICD10), data interoperability (FHIR), diagnosis code (SNOMED CT), to workflow in clinical decision support systems. Then, it dives deep into how AI innovations are transforming our healthcare system by focusing on AI in drug discovery, AI in medical image diagnosis, explainable AI for health risk prediction, and ethics of AI in healthcare.
What You Will Learn
- Be aware of current healthcare initiatives to deliver quality care
- Understand the technologies underlying health IT systems, including data semantics, data interoperability, workflow, and clinical decision support systems
- Deepen understanding of electronic health record systems (EHR systems)
- Gain a broad overview of AI innovations in healthcare
- Master practical skills of data search and analytics including database search, natural language processing, data visualization, machine learning, and deep learning
Syllabus
- Evidence-based Care, i2b2 and OMOP
- EMR Semantics: ICD10, ICD10 (COVID) and ICD9 (MIMIC)
- EMR Semantics: SNOMED CT I
- EMR Semantics: SNOMED CT II, SNOMED and ICD10
- EMR Semantics: LOINC
- EMR Semantics: RxNorm
- Clinical Decision Support System
- Data Share: FHIR
- AI health: ML/DL I (Explainable AI and Multimodal fusion learning)
- AI health: ML/DL II Advanced Medical NLP
- AI health: imaging (Medical Imaging Diagnosis)
- AI in Drug Discovery
- Ethics of AI in Health
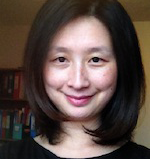
Bill & Lewis Suit Professor, School of Information
This is a course on computational logic and its applications in computer science, particularly in the context of software verification. Computational logic is a fundamental part of many areas of computer science, including artificial intelligence and programming languages. This class introduces the fundamentals of computational logic and investigates its many applications in computer science. Specifically, the course covers a variety of widely used logical theories and looks at algorithms for determining satisfiability in these logics as well as their applications.
Syllabus
- Normal forms; decision procedures for propositional logic; SAT solvers (2 weeks)
- Applications of SAT solvers and binary decision diagrams (1 week)
- Semantics of to first-order logic and theoretical properties (1 weeks)
- First-order theorem proving (1.5 weeks)
- Intro to first-order theories (0.5 week)
- Theory of equality (0.5 week)
- Decision procedures for rationals and integers (1.5 weeks)
- DPLL(T) framework and SMT solvers (1 week)
- Basics of software verification (1 week)
- Automating software verification (2 weeks)
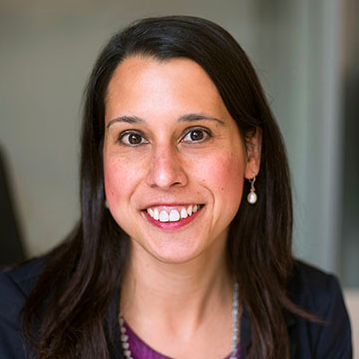
Professor, Computer Science
The Case Studies in Machine Learning course presents a broad introduction to the principles and paradigms underlying machine learning, including presentations of its main approaches, overviews of its most important research themes and new challenges faced by traditional machine learning methods. This course highlights major concepts, techniques, algorithms, and applications in machine learning, from topics such as supervised and unsupervised learning to major recent applications in housing market analysis and transportation. Through this course, students will gain experience by using machine learning methods and developing solutions for a real-world data analysis problems from practical case studies.
What You Will Learn
- Understand generic machine learning (ML) terminology
- Understand motivation and functioning of the most common types of ML methods
- Understand how to correctly prepare datasets for ML use
- Understand the distinction between supervised and unsupervised learning, as well the interests and difficulties of both approaches
- Practice script implementation (Python/R) of different ML concepts and algorithms covered in the course
- Apply software, interpret results, and iteratively refine and tune supervised ML models to solve a diverse set of problems on real-world datasets
- Understand and discuss the contents and contributions of important papers in the ML field
- Apply ML methods to solve real world problems and present them to mini clients
- Write reports in which results are assessed and summarized in relation to aims, methods and available data
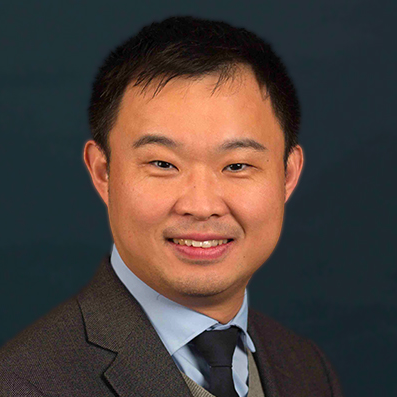
Associate Professor, School of Architecture
This course focuses on modern natural language processing using statistical methods and deep learning. Problems addressed include syntactic and semantic analysis of text as well as applications such as sentiment analysis, question answering, and machine translation. Machine learning concepts covered include binary and multiclass classification, sequence tagging, feedforward, recurrent, and self-attentive neural networks, and pre-training / transfer learning.
What You Will Learn
- Linguistics fundamentals: syntax, lexical and distributional semantics, compositional semantics
- Machine learning models for NLP: classifiers, sequence taggers, deep learning models
- Knowledge of how to apply ML techniques to real NLP tasks
Syllabus
- ML fundamentals, linear classification, sentiment analysis (1.5 weeks)
- Neural classification and word embeddings (1 week)
- RNNs, language modeling, and pre-training basics (1 week)
- Tagging with sequence models: Hidden Markov Models and Conditional Random Fields (1 week)
- Syntactic parsing: constituency and dependency parsing, models, and inference (1.5 weeks)
- Language modeling revisited (1 week)
- Question answering and semantics (1.5 weeks)
- Machine translation (1.5 weeks)
- BERT and modern pre-training (1 week)
- Applications: summarization, dialogue, etc. (1-1.5 weeks)
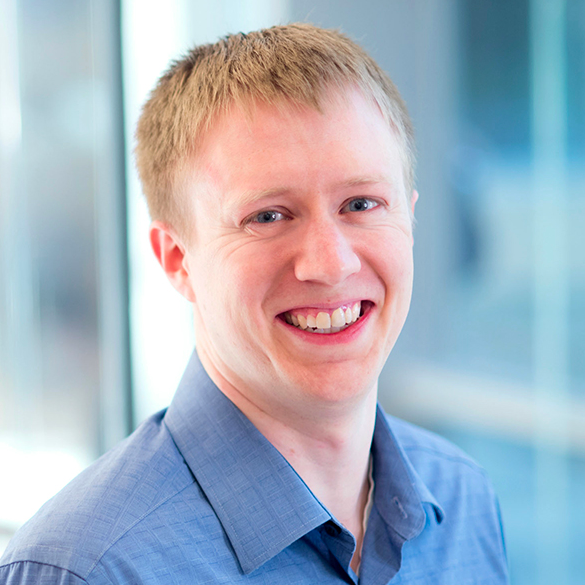
Assistant Professor, Computer Science
This class has two major themes: algorithms for convex optimization and algorithms for online learning. The first part of the course will focus on algorithms for large scale convex optimization. A particular focus of this development will be for problems in Machine Learning, and this will be emphasized in the lectures, as well as in the problem sets. The second half of the course will then turn to applications of these ideas to online learning.
What You Will Learn
- Techniques for convex optimization such as gradient descent and its variants
- Algorithms for online learning such as follow the leader and weighted majority
- Multi-Armed Bandit problem and its variants
Syllabus
- Convex sets and Convex functions, including basic definitions of convexity, smoothness and strong convexity
- First order optimality conditions for unconstrained and constrained convex optimization problems
- Gradient and subgradient descent: Lipschitz functions, Smooth functions, Smooth and Strongly Convex functions
- Oracle Lower Bounds
- Accelerated Gradient Methods
- Proximal and projected gradient descent. ISTA and FISTA
- Mirror Descent
- Frank Wolfe
- Stochastic Gradient Descent
- Stochastic bandits with finite number of arms: Explore and commit algorithm, UCB algorithm and regret analysis
- Adversarial bandits with finite number of arms: Exponential weighting and importance sampling, Exp3 algorithm and variants
- Multi-armed Bandit (MAB) lower bounds: minimax bounds, problem-dependent bounds
- Contextual bandits: Bandits with experts — the Exp4 algorithm, stochastic linear bandits, UCB algorithm with confidence balls (LinUCB and variants)
- Contextual bandits in the adversarial setting: Online linear optimization (with full and bandit feedback), Follow The Leader (FTL) and Follow the Regularized Leader (FTRL), Mirror Descent
- Online Classification: Halfing algorithm, Weighted majority algorithm, Perceptron and Winnow algorithms (with connections to Online Gradient Descent and Online Mirror Descent)
- Other Topics: Combinatorial bandits, Bandits for pure exploration, Bandits in a Bayesian setting, Thompson sampling
- Newton and Quasi-Newton Methods
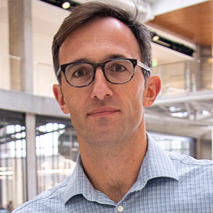
Professor, Electrical & Computer Engineering
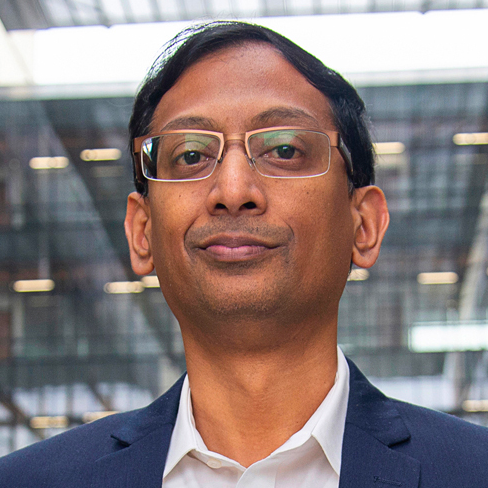
Professor, Electrical & Computer Engineering
This class covers linear programming and convex optimization. These are fundamental conceptual and algorithmic building blocks for applications across science and engineering. Indeed any time a problem can be cast as one of maximizing / minimizing and objective subject to constraints, the next step is to use a method from linear or convex optimization. Covered topics include formulation and geometry of LPs, duality and min-max, primal and dual algorithms for solving LPs, Second-order cone programming (SOCP) and semidefinite programming (SDP), unconstrained convex optimization and its algorithms: gradient descent and the newton method, constrained convex optimization, duality, variants of gradient descent (stochastic, subgradient etc.) and their rates of convergence, momentum methods.
Syllabus
- Convex sets, convex functions, Convex Programs (1 week)
- Linear Programs (LPs), Geometry of LPs, Duality in LPs (1 week)
- Weak duality, Strong duality, Complementary slackness (1 week)
- LP duality: Robust Linear Programming, Two person 0-sum games, Max-flow min-cut (1 week)
- Semidefinite programming, Duality in convex programs, Strong duality (1 week)
- Duality and Sensitivity, KKT Conditions, Convex Duality Examples: Maximum Entropy (1 week)
- Convex Duality: SVMs and the Kernel Trick, Convex conjugates, Gradient descent (1 week)
- Line search, Gradient Descent: Convergence rate and step size, Gradient descent and strong convexity (1 week)
- Frank Wolfe method, Coordinate descent, Subgradients (1 week)
- Subgradient descent, Proximal gradient descent, Newton method (1 week)
- Newton method convergence, Quasi-newton methods, Barrier method (1 week)
- Accelerated Gradient descent, Stochastic gradient descent (SGD), Mini-batch SGD, Variance reduction in SGD (1 week)
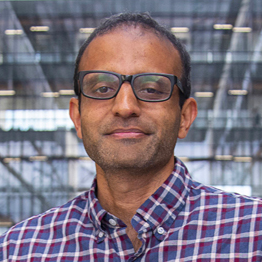
Associate Professor, Electrical and Computer Engineering
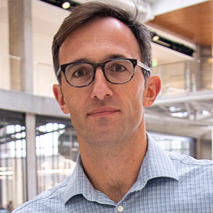
Professor, Electrical & Computer Engineering
Important Dates
Fall Application
Spring Application
Please note: Applying to UT Austin is a twofold process. We recommend that applicants apply to UT Austin before the priority deadline. This is to ensure their materials are processed in a timely manner.